5 Ways To Utilize The Immense Power of Large Language Models In Finance
Investor Alexander Voigt shares his perspectives and insights about the potential of large language models in finance.
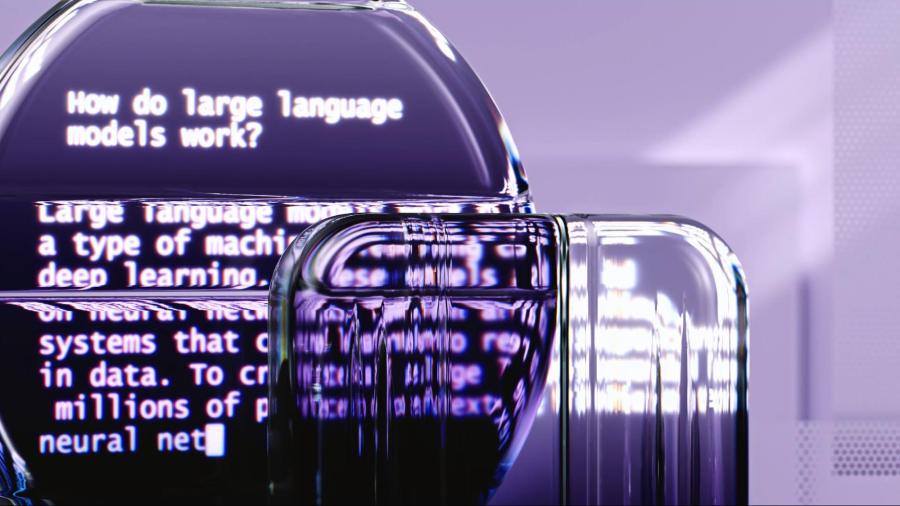
Presented by Tom White
An AI gold rush has just begun. There are new business opportunities in an ever-changing world where a Large Language Model (LLM) can transform jobs and disrupt multiple industries. Whether within large corporations or startups, not a month goes by without news about new LLMs and tools. Based on the nature of LLMs, the most influenced sectors are those using data, including the finance sector.
Below, investor Alexander Voigt shares his perspectives and insights about the potential benefits of LLM utilization in the finance sector.
Analyzing Stock Market Data
LLMs can generate investing ideas by gathering stock market data from leading stock analysis websites. Thousands of new stock market news articles, earnings reports and analyst estimates are published daily, which can be challenging for a single person to extract specific pieces of information from.
Using Large Language Models helps reduce the whole information set to the central elements. The structured data can be used to understand a company’s market position, growth potential, and financial health more clearly. The data can also identify trends, market sentiments and trading patterns.
Researching Economic Trends
Large Language Models help analysts to research economic trends more intensively by utilizing historical data to predict economic trends. Analyzing such data has been done before the rise of Large Language Models, but not to that extent. LLM tools access the entire internet and collect economic data from all over the world.
An example use case is predicting the interest rates of central banks, like the Federal Reserve, based on historical data in dependence on other economic-relevant data points. LLMs can, for example, use historical correlation to estimate how the interest rates will change if specific criteria are met, where relevant comparison criteria can be unemployment rates, GDP growth and inflation.
Preparing Financial Reports
Report generation is a time-consuming and repetitive task in finance. Companies typically have style guides for their financial reports. Still, how the data is gathered, processed and visualized can differ from department to department. LLM tools help to structure the process more clearly and prepare financial reports in an efficient way.
The data will help a company’s CFO strengthen their position by enabling them to make well-informed decisions based on precise financial data. AI is not limited to collecting and structuring data. It is also possible to use AI to summarize key points, observations or trends identified in structured financial data.
Structuring Data
Unstructured data is a no-go in the financial industry because it costs too much in human resources and leads to incorrect interpretations and conclusions. Consider a situation where a parent company collects data monthly from its subsidiaries without clear guidance on structuring the data. Reports can be checked, restructured and summarized by hand, but defining a clear structure is more efficient.
LLM-based software can be used to define such a structured data template by gathering all the information from all reports available and combining it into a central template that captures all data correctly.
Analyzing And Structuring Raw Data
Thirty years ago, newspapers were the primary source of information. To gather as much data as possible, it was necessary to buy multiple newspapers and read all of them. Only the person who read all articles could combine all the data to make a conclusion and derive appropriate measures.
Times have changed, and today, there is an apparent surplus of information, where tools like Large Language Models help to gain back control of data analysis.
With its access to all information available online, Large Language Models help collect an immense amount of raw data. At first, the data is typically unstructured, but the LLM converts it into an appropriate structure, making it possible to process it further. In the second step, LLM tools analyze the data and reduce it to the essence of the whole.
Benefits of Using Large Language Models In Finance
Improve Productivity
LLMs can be used for automatically analyzing a high amount of data points in a structured way. In addition, using LLMs for report generation and financial modeling saves a tremendous amount of time, which improves productivity.
Answer Questions
Answering questions takes a lot of time, and it’s often a repetitive process. Questions that relate to a specific topic can be asked in different ways, whereas answers can also be multifold, even if the core element of a question is the same. LLMs help to analyze the central component of a question and can automatically answer it in a pre-defined way.
Discover New Analysis Processes
Process optimization can save a company time, money and resources, but it is a tremendous task with high complexity. LLMs reduce complexity and analyze processes in a structured way.
Disadvantages of Using Large Language Models in Finance
Requires Training
LLMs are only as good as their training models and rely on available data. This holds true for how the developer algorithmically trains the overall system and how the end-user trains the tool while using it.
Risk of Inaccurate Information
The system can produce inaccurate information and errors if a task is too complex or too much data is processed. If those so-called hallucinations happen, it’s typically required to be more specific about a request.
Human Fact-Checking Needed
LLMs cannot check if the information found on the internet is correct. Suppose multiple sources cite a specific number repeatedly. In that case, LLMs will likely conclude that the data found most frequently is right, even if it is wrong.